A Machine Learning Framework for Quantifying Chemical Segregation and Microstructural Features in Atom Probe Tomography Data
New publication
2023/11/13
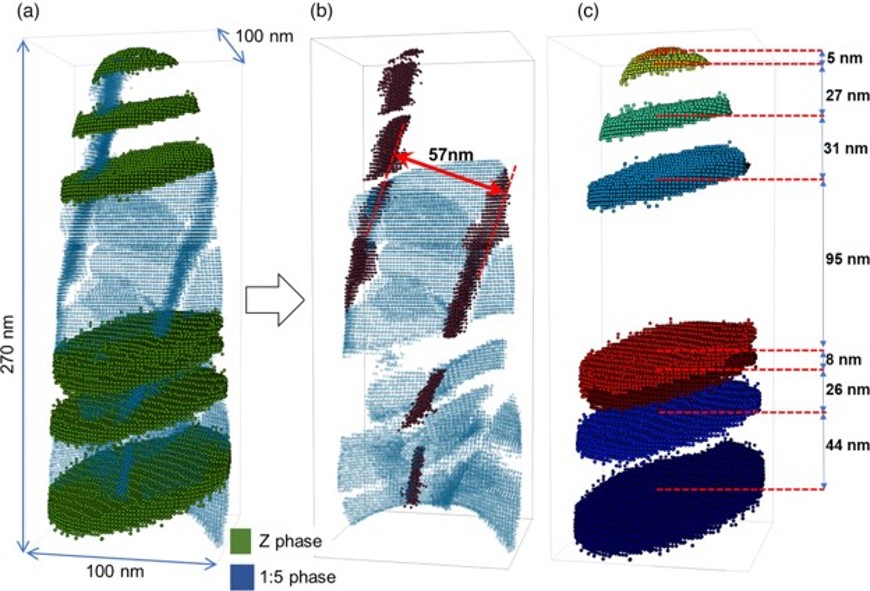
Atom probe tomography (APT) is ideally suited to characterize and understand the interplay of segregation and microstructure in modern multicomponent materials. Yet, the quantitative analysis typically relies on human expertise to define regions of interest. We introduce a computationally efficient, multi-stage machine learning strategy to identify compositionally distinct domains in a semi-automated way, and subsequently quantify their geometric and compositional characteristics. In our algorithmic pipeline, we first coarse-grain the APT data into voxels, collect the composition statistics, and decompose it via clustering in composition space. The composition classification then enables the real-space segmentation via a density-based clustering algorithm, thus revealing the microstructure at voxel resolution. Our approach is demonstrated for a Sm–(Co,Fe)–Zr–Cu alloy. The alloy exhibits two precipitate phases with a plate-like, but intertwined morphology. The primary segmentation is further refined to disentangle these geometrically complex precipitates into individual plate-like parts by an unsupervised approach based on principle component analysis, or a U-Net-based semantic segmentation trained on the former. Following the composition and geometric analysis, detailed composition distribution and segregation effects relative to the predominant plate-like geometry can be readily mapped from the point cloud, without resorting to the voxel compositions.
A. Saxena , N. Polin, N. Kusampudi , S. Katnagallu, L. Molina-Luna, O. Gutfleisch, B. Berkels, B. Gault, J. Neugebauer, and C. Freysoldt
Microscopy and Microanalysis, 29, 1658–1670 (2023).
DOI: 10.1093/micmic/ozad086
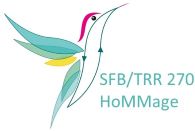