AI-Driven Design of RNA-based Sensors for In-Vitro Diagnostics
Goal of the Project:
The goal is to design synthetic intrinsic terminators that respond to specific biomarker small RNAs. These terminators should exhibit controlled transcription termination and switchability based on external RNA signals. The project will use computational tools, AI, and experimental data to develop and refine these terminators. By combining AI with established knowledge of sequence requirements, thermodynamics, and medium-throughput screening, we aim to develop highly efficient and responsive terminator systems.
Methodology:
1. Analyze Natural Terminators: Utilize an intrinsic terminator database to identify key sequence and structural features. These features will guide the design of synthetic terminators.
2. Predict Folding with NUPACK: Use NUPACK to model how synthetic terminator sequences fold and how their interactions with small RNAs can either stabilize or disrupt termination. This step ensures designs are thermodynamically feasible.
3. Integrate Known Small RNA-Targeting Designs: Build upon the reported 100+ designs in the literature, refining design rules that can be generalized to other RNA structures.
4. AI-Powered Design and Iteration:
• Initial Training: Train the model using known terminator datasets, thermodynamic predictions, and supplementary material from published work to extract function–sequence relationships.
• Feedback Loop: After experimental testing, feed data on terminator efficiency, leakiness, and switchability back into the model to refine future designs (possibly beyond the scope of this thesis, depending on timing).
5. Design for Biomarker Small RNAs: Use a curated list of biomarker small RNAs as inputs to design terminators that respond specifically to these molecules. This ensures practical applications in biosensors or dynamic gene circuits.
6. Experimental Validation (performed by collaborators): Test the predicted sequences in the lab to measure termination efficiency, response to external small RNAs, and potential leakiness. This data will validate the AI predictions and inform subsequent design cycles.
Expected Outcome:
You will deliver synthetic terminators that are switchable, minimally leaky, and responsive to specific small RNAs. By integrating experimental feedback into an AI-driven DBTL framework, these designs will improve iteratively, demonstrating the power of combining computational tools with experimental biology. The project offers hands-on experience with bioinformatics, thermodynamic modeling, and machine learning, preparing you for advanced research or industrial applications in synthetic biology and artificial intelligence.
Prerequisites:
• Background in medical engineering, electrical engineering, computer science, (computational) biology, mathematics, physics, or related fields.
• Familiarity with Python and machine learning frameworks (e.g., TensorFlow, PyTorch).
• Knowledge of RNA biology is helpful but not mandatory; an introduction will be provided.
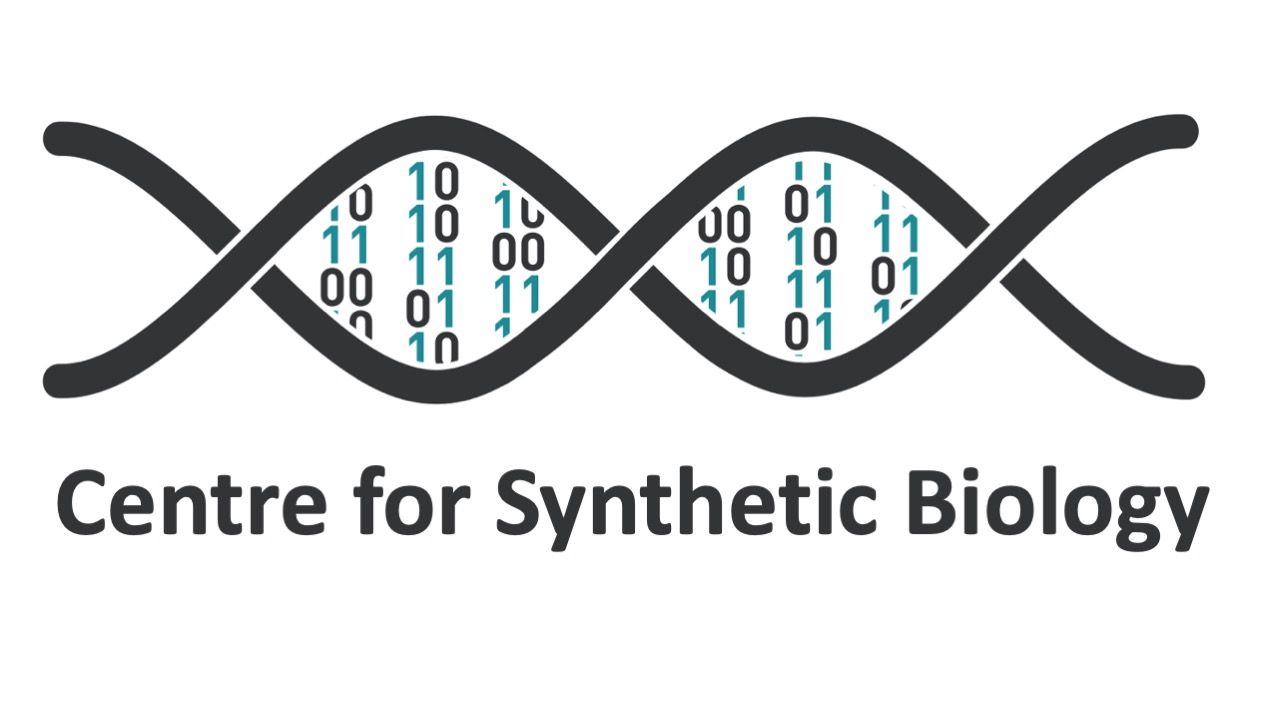