Novembertalks on AI, ML & Robotics
2020/10/29
29.10.2020, 14:00-15:00 h
Gergely Neu, Universitat Pompeu Fabra: Logistic Q-Learning
05.11.2020, 14:00-15:00 h
Emtiyaz Khan, RIKEN Advanced Intelligence Project: Bayesian Principles for Learning Machines
12.11.2020, 15:00-16:00 h
Nathan Ratliff, NVIDIA AI Lab: Geometric fabrics: Transparent tools for behavior engineering
19.11.2020, 14:00-15:00 h
Zico Kolter, CMU: Equilibrium approaches to deep learning: One (implicit) layer is all you need
Please register at in advance to receive a Zoom link for participation. http://tiny.cc/7askoz
Abstracts and bios:
==============================
Title: Logistic Q-Learning
Presenter: Gergely Neu, Universitat Pompeu Fabra
Date & Time: 29.10.2020, 14:00-15:00 h
Abstract:
This talk will be a highly technical talk on a new reinforcement learning algorithm derived from a regularized linear programming formulation of optimal control in MDPs. The method is closely related to the classic Relative Entropy Policy Search (REPS) algorithm — with the key difference that our method introduces a Q-function that enables efficient exact model-free implementation. The main feature of our algorithm (called Q-REPS) is a convex loss function for policy evaluation that serves as a theoretically sound alternative to the widely used squared Bellman error. We provide a practical saddle-point optimization method for minimizing this loss function and provide an error-propagation analysis that relates the quality of the individual updates to the performance of the output policy. Finally, we demonstrate the effectiveness of our method on a range of benchmark problems.
Bio:
Gergely Neu is a leading researcher on the theoretical and mathematical foundations of artificial intelligence in particular for reinforcement learning and an assistant professor at Universitat Pompeu Fabra at Universitat Pompeu Fabra. He received a PhD on Online learning in non-stationary Markov decision processes working with András György (MTA SZTAKI, Hungary), Csaba Szepesvári (University of Alberta, Canada) and László Györfi (Budapest University of Technology and Economics, Hungary). He has been a postdoctoral researcher at the SequeL team at INRIA Lille as well as a visiting researcher at the Simons Institute for the Theory of Reinforcement Learning at Berkeley CA, USA, at University of Alberta, Edmonton AB, Canada and at Google Brain.
He has received numerous prestigious awards and grants, most recently an ERC Starting Grant.
==============================
Title: Bayesian Principles for Learning Machines
Presenter: Emtiyaz Khan, RIKEN Advanced Intelligence Project
Date & Time: 05.11.2020, 14:00-15:00 h
Abstract:
Humans and animals have a natural ability to autonomously learn and quickly adapt to their surroundings. How can we design machines that do the same? In this talk, I will present Bayesian principles to bridge such gaps between humans and machines. I will show that a wide-variety of machine-learning algorithms are instances of a single learning-rule derived from Bayesian principles. The rule unravels a dual-perspective yielding new mechanisms for knowledge transfer in learning machines. My hope is to convince the audience that Bayesian principles are indispensable for an AI that learns as efficiently as we do.
Bio:
Emtiyaz Khan (also known as Emti) is a team leader at the RIKEN center for Advanced Intelligence Project (AIP) in Tokyo where he leads the Approximate Bayesian Inference Team. He is also a visiting professor at the Tokyo University of Agriculture and Technology (TUAT). Previously, he was a postdoc and then a scientist at Ecole Polytechnique Fédérale de Lausanne (EPFL), where he also taught two large machine learning courses and received a teaching award. He finished his PhD in machine learning from University of British Columbia in 2012. The main goal of Emti’s research is to understand the principles of learning from data and use them to develop algorithms that can learn like living beings.
For the past 10 years, his work has focused on developing Bayesian methods that could lead to such fundamental principles. The approximate Bayesian inference team now continues to use these principles, as well as derive new ones, to solve real-world problems.
==============================
Title: Geometric fabrics: Transparent tools for behavior engineering
Presenter: Nathan Ratliff, NVIDIA AI Lab
Date & Time: 12.11.2020, 15:00-16:00 h
Abstract:
Industry tends to shy away from promising new learning-based tools in favor of the well-understood model-based planners and controllers engineers are comfortable employing on mission critical problems. The importance of deep learning in robotics is, of course, real; we will never achieve proficient perception-driven behavior on human-interactive problems without data driven approaches. But this hesitation holds
water: engineers can’t engineer on excitement alone. Deep data driven approaches will remain inappropriate for many industrial applications until we understand them as well as we understand the planners and controllers they aim to replace. In this talk, I will present a new mathematical framework for behavioral engineering called optimization fabrics designed to bridge this gap. The theory of optimization fabrics gives rise to a concrete toolset for behavioral design called geometric fabrics which enable the flexible and modular construction of intelligent behaviors, including smooth obstacle avoidance subsystems, with well-understood stability properties and intuitive composability.
Our long-term goal is to add transparency to the design of the types of behavioral systems we want to build into deep learning architectures with an eye toward robust generalization. Toward that goal, at NVIDIA geometric fabrics have enabled us to hand-engineer a number of strongly generalizing real-world system demos. Throughout the talk, I will show a number of these systems and present some of our newest theoretical and experimental results.
Bio:
Nathan is a senior researcher at NVIDIA AI Lab at Seattle, WA, USA. He has been a researcher at TTI-C on the University of Chicago Campus building robots, Intel Labs in both Seattle and Pittsburgh studying trajectory optimization, at the MPI for Intelligent Systems, and Google developing large scale learning systems to assess the quality of Ad Landing Pages. He earned my PhD from Carnegie Mellon's Robotics Institute in 2009 studying imitation learning, structured prediction, and functional gradient techniques for learning and optimization. Drew Bagnell, Martin Zinkevich, and I developed a methodology for training planners and control algorithms for robotics (Inverse Optimal Control
(IOC)) using ideas from Maximum Margin Structured Classification (MMSC).
Our framework is known as Maximum Margin Planning (MMP); we developed a family of online, batch, and functional subgradient methods (exponentiated boosting), collectively known as LEArning to seaRCH to learn efficiently within the framework. Applications include footstep prediction, grasp prediction, heuristic learning, overhead navigation, LADAR classification, and optical character recognition.
==============================
Title: Equilibrium approaches to deep learning: One (implicit) layer is all you need
Presenter: Zico Kolter, CMU
Date & Time: 19.11.2020, 14:00-15:00 h
Abstract:
Does deep learning actually need to be deep? In this talk, I will present some of our recent and ongoing work on Deep Equilibrium (DEQ) Models, an approach that demonstrates we can achieve most of the benefits of modern deep learning systems using very shallow models, but ones which are defined implicitly via finding a fixed point of a nonlinear dynamical system. I will show that these methods can achieve results on par with the state of the art in domains spanning large-scale language modeling, image classification, and semantic segmentation, while requiring less memory and simplifying architectures substantially. I will also highlight some recent work analyzing the theoretical properties of these systems, where we show that certain classes of DEQ models are guaranteed to have a unique fixed point, easily-controlled Lipschitz constants, and efficient algorithms for finding the equilibria. I will conclude by discussing ongoing work and future directions for these classes of models.
Bio:
Zico Kolter is an Associate Professor in the Computer Science Department at Carnegie Mellon University, and also serves as chief scientist of AI research for the Bosch Center for Artificial Intelligence. His work spans the intersection of machine learning and optimization, with a large focus on developing more robust and rigorous methods in deep learning. In addition, he has worked in a number of application areas, highlighted by work on sustainability and smart energy systems. He is a recipient of the DARPA Young Faculty Award, a Sloan Fellowship, and best paper awards at NeurIPS, ICML (honorable mention), IJCAI, KDD, and PESGM.
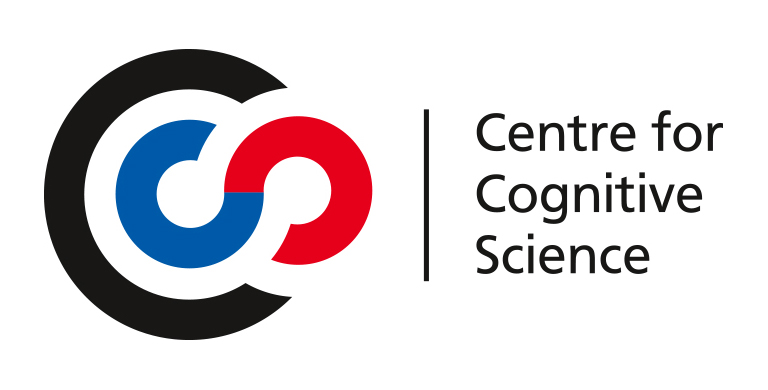